Insurance AI Data Extraction: How to Find the Right Vendor and Where to be Cautious
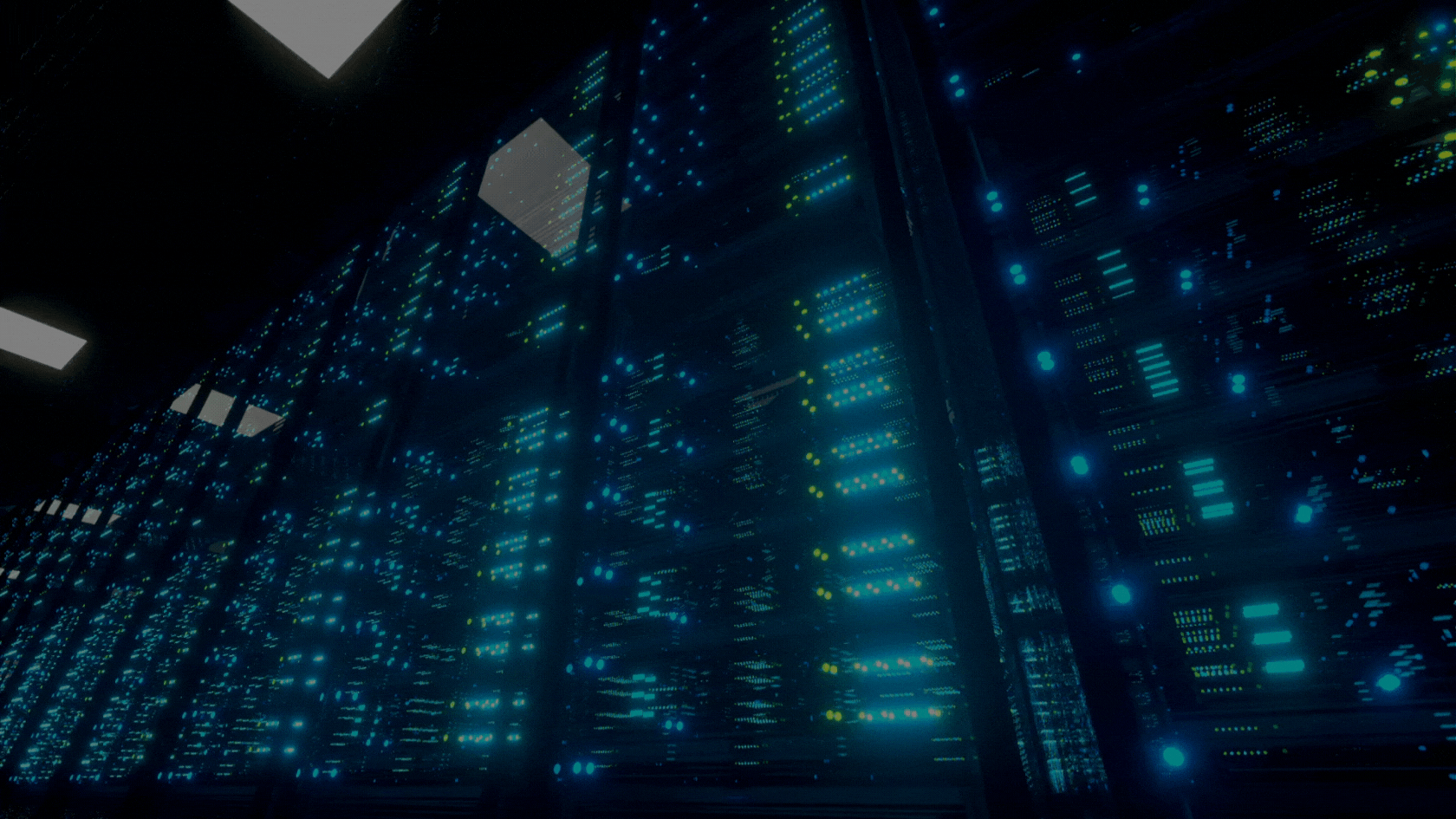
Navigating the Insurance AI Data Extraction Market
In a busy market filled with Gen AI vendors, all saying similar things around Insurance AI data extraction, it can be very difficult to figure out which ones are the real deal and where to invest time to test.
As the excitement around AI grows, (re)Insurers, MGAs, and Brokers, are faced with lots of choices. The growing market experience is that many vendors don’t live up to their promises, projects take months and years, never ending cycles of trying to increase AI accuracy, never ending costs.
Narrow the field
- Look for Insurance AI Data Extraction vendors with clients publicly sharing positive recent experiences. Be cautious if there are no such endorsements. It’s crucial that these clients are at least partway through implementations or even better have fully deployed. Furthermore, proving that the vendor’s solutions work in a real-world setting.
- Avoid getting caught up in clever sounding buzzwords. Because the complexity of AI often results in overlapping terms which do not mean that much.
- Does the vendor advertise information security accreditations relevant for your business? if not, the vendor probably does not fully understand your business.
- Seek vendors who understand the nuances of your actual business and specific business lines. You can test them on this in a meeting. A vendor having expertise in one area, like invoice extraction, doesn’t necessarily translate to proficiency in insurance submission extraction. The field/entity level of detail in underwriting or claims terms needs to be well understood.
- Will the vendor commercially stand behind data extraction accuracy claims?
- Does the vendor have a credible and believable set of pre-trained AI models?
Testing the Vendor
Here are some vendors you can vet yourself to identify the best match tailored to your business needs. This report is collated by Datos Insights*.
- Once you identify the right matches, it’s crucial to run a test. When testing Insurance AI Data Extraction vendors, go beyond typical assessments and focus on the most complex use cases. Complexity is more critical than volume. Certainly, scalable infrastructure can very easily deal with volume. Indeed, complexity of use cases is much harder and will test the potential vendor.
- Clearly define your business case and prioritize testing against those parameters. For example, are property Schedules are a key part of your business case, test your most complex ones.
- Watch out for ‘bells and whistles’ that look cool but are not part of your business case. Either update your business case with benefits attached to these features or ignore them.
- Fairness is key – the vendor will require set up time, a few days usually, but a vendor who understands your business should not need more than 1 or 2 examples of your data to be able to execute a test, if there is a request for lots of data or AI training time, probably the vendor does not understand your business and what the AI needs to be extracting.
- Vendors that need multiple weeks to setup their platform for a POC either have capacity bottlenecks or will not realistically be able to support accelerated deployment at scale.
Beware, the test is not everything. There are many examples of vendors who have passed initial tests but are then removed by the client after a frustrating 6-18 months of an unsuccessful expensive implementation. This leads us to a critical point that is often missed until it is too late. Can the vendor deploy at scale?
Deployment is usually not focused on, until it is too late
Deployment capability is a major market issue, especially at scale. In a proof of concept, especially a free one, testing the vendors ability to deploy is challenging.
Furthermore, we all know that the faster we can deploy, the faster the project is over, the faster the benefits are achieved.
To avoid this gotcha,
- Devote attention to understanding how well the vendor grasps the specifics of your business lines, as you know, the devil is in the detail.
- Request to speak to one of the vendors clients about their rollout experiences with the vendor, ideally a client who is well past deployment and is using the tool successfully in their day-to-day operations. Be clear on the questions you ask the client to really understand where they are in the process of deployment. Focus on how fast they got to extracting data at the accuracy level required for their business.
- Deployment cost is key to your business case. Get clarity on the activities that you as the client are responsible for and ensure that the cost of such activities is reflected in the vendor TCO (Total Cost of Ownership).
- Think about the complexities of your business, the different data fields that require extracting for the different lines of business and languages. Speak to the vendor and question them on how they would deal with these challenges. Do they understand the detail of what needs extracting and the context, if so how is it factored in their deployment plan.
- Additionally, do they stand behind the deployment plan and extraction accuracy commercially, i.e., they get paid if it works, not if it doesn’t work?
- Vendors who do not have a track record of deployment likely need a strong Consultancy/Professional Services partner, especially if you want to scale across your business. Look for vendors who are open to these partnerships and actively seek references from the partners.
Pricing
- In the light of evaluating pricing, be cautious of ‘AI training’ in time and materials deployment plans. Equally, be wary of fixed-price deployments laden with numerous caveats.
- Target vendors with a proven track record in similar businesses and a team that demonstrates deep insurance understanding, ideally with members who have worked within insurance companies.
- Actively look for vendors that commercially stand behind their extraction accuracy and deployment speed claims.
- Furthermore, avoid vendors with commercial models not linked to value you’re extracting from the platform e.g. If you are looking for data fields extracted, this is the value. If you are looking for pages read by the machine, this is the value. If you are looking for risks triaged, this is the value.
Critical Self-Reflection questions
- Does the extraction accuracy look as we need in the testing?
- Can the vendor deploy at the pace we need? Have they demonstrated this with client reference calls?
- Does the vendor possess the understanding of our business? Or are we going to need to teach them?
- Lastly, ensure the TCO calculation includes both software costs and implementation effort estimates across all the major LOBs and process steps to be automated.
- Are the implementation efforts based on vendor estimates or an expert, experienced view?
Watch this space for our next article on – Implementing successfully data extraction with Gen AI
Upcoming Content
See our existing articles on,
- Beware of the risks surrounding AI training
- Industry leaders views on Insurance data extraction, the pitfalls and successes
*Datos Insights
Datos Insights delivers the most comprehensive and industry-specific data and advice to the companies trusted to protect and grow the world’s assets, and to the technology and service providers who support them. Staffed by experienced industry executives, researchers, and consultants, we support the world’s most progressive banks, insurers, investment firms, and technology companies. Generally, advisory subscriptions, data services, custom projects, consulting, conferences, and executive councils this is do.